AI-powered underwriting is transforming how insurance companies assess risk. It uses powerful algorithms and vast datasets to automate the underwriting process, potentially leading to faster decisions and improved accuracy. This new approach offers a more efficient and potentially fairer way to evaluate risk, but also brings new challenges related to data bias and regulatory compliance. This overview will explore the exciting possibilities and the hurdles of this innovative technology.
Traditional underwriting relies heavily on human judgment, which can be prone to bias. AI-powered underwriting promises to reduce this bias by analyzing data in a more objective way. By processing massive amounts of data, AI can identify patterns and insights that humans might miss, leading to more accurate risk assessments and potentially lower premiums for qualified applicants.
Introduction to AI-powered Underwriting
AI-powered underwriting is revolutionizing the insurance industry by leveraging artificial intelligence to automate and enhance the traditional underwriting process. It analyzes vast amounts of data to assess risk more accurately and efficiently, ultimately leading to better decisions and improved customer outcomes.This new approach utilizes sophisticated algorithms and machine learning models to make faster and more objective underwriting decisions. By incorporating a broader range of data sources, AI can identify subtle patterns and insights that might be missed by human underwriters, resulting in a more comprehensive and data-driven risk assessment.
Definition of AI-powered Underwriting
AI-powered underwriting employs artificial intelligence (AI) techniques to automate and enhance the process of evaluating risk for insurance policies. It involves using algorithms and machine learning models to analyze data, predict outcomes, and make underwriting decisions. This contrasts with traditional underwriting, which relies heavily on human judgment and limited data sets.
Fundamental Principles of AI in Underwriting
The core principles behind using AI in underwriting hinge on leveraging data to improve decision-making. AI models are trained on large datasets containing historical underwriting information, along with external factors like socioeconomic indicators and behavioral data. This allows the models to identify patterns and correlations that might not be apparent to human underwriters. These models then use this knowledge to predict future risk, helping insurers price policies more accurately and efficiently.
AI Technologies Used in Underwriting
A variety of AI technologies are being implemented in underwriting, each playing a specific role in the process. Machine learning, a subset of AI, is frequently used to build predictive models that analyze vast amounts of data to identify patterns and make risk assessments. Deep learning, another powerful machine learning technique, can analyze complex and unstructured data, like social media posts or images, to extract valuable insights for underwriting.
Natural Language Processing (NLP) allows the system to interpret and understand text data, such as customer communications or policy documents, further enriching the data used for analysis.
Historical Context of Underwriting and the Impact of AI
Traditional underwriting has historically relied on human judgment, experience, and limited data sets. The process was often time-consuming, prone to bias, and less accurate than it could be. AI-powered underwriting represents a significant shift, automating many aspects of the process, including data analysis, risk assessment, and decision-making. This automation has the potential to reduce processing time, improve accuracy, and increase efficiency.
Traditional vs. AI-Powered Underwriting
Feature | Traditional Underwriting | AI-Powered Underwriting |
---|---|---|
Data Source | Limited, primarily from historical data and basic customer information | Extensive, encompassing diverse data points like social media activity, online behavior, and credit scores. |
Decision-Making | Based on human judgment and experience | Automated, using algorithms and predictive models |
Speed | Slower, often requiring multiple steps and approvals | Faster, enabling quicker processing and turnaround times |
Accuracy | Prone to human bias and inconsistencies | Potentially more objective, minimizing human error and increasing accuracy. |
Benefits of AI in Underwriting
AI is rapidly transforming the underwriting process, bringing a host of advantages that promise to reshape how insurance companies assess risk and manage their operations. These benefits extend beyond simple automation, impacting the very core of decision-making and potentially reducing bias. The ability to analyze vast amounts of data and identify intricate patterns gives AI a powerful edge in underwriting.
Improved Efficiency and Reduced Costs
AI-powered underwriting systems can significantly speed up the decision-making process. Instead of relying on manual reviews, AI algorithms can analyze applications and relevant data points much faster, potentially cutting processing time by a substantial margin. This acceleration translates into reduced turnaround times for policy approvals, a crucial factor in attracting and retaining customers. Furthermore, automation reduces the need for a large human workforce, thereby decreasing operational costs associated with salaries and administrative overhead.
By streamlining processes and minimizing manual errors, AI contributes to a more efficient and cost-effective underwriting operation.
Increased Accuracy and Reduced Bias
AI algorithms can analyze a wider range of data points than humans, potentially leading to a more comprehensive and accurate assessment of risk. This includes factors that might be overlooked in traditional methods, like subtle patterns in applicant behavior or historical claims data. By incorporating a vast dataset, AI can identify correlations and patterns that might indicate a higher or lower risk profile, leading to more informed and potentially more accurate underwriting decisions.
This increased accuracy can lead to a more robust risk management strategy, and critically, can potentially mitigate bias. By removing human subjectivity, AI-powered systems can reduce the likelihood of discriminatory practices based on factors like race, gender, or socioeconomic status. This objectivity can enhance fairness and lead to a more equitable insurance market.
AI-powered underwriting is changing how businesses assess risk. This often involves streamlining the process, which is where mobile payments come in handy. Mobile payments are becoming increasingly popular, making it easier for companies to collect and verify payment information, which in turn allows for more efficient and accurate underwriting. This ultimately leads to faster loan approvals and better risk management for AI-powered underwriting systems.
Enhanced Risk Assessment and Fraud Detection
AI’s ability to process large datasets makes it exceptionally effective in identifying complex risk factors and fraudulent activities. Sophisticated algorithms can analyze historical claims data, financial records, and even social media activity to identify potential risks or fraudulent claims. This proactive approach to risk assessment allows insurance companies to better anticipate and manage potential losses, and through fraud detection, they can prevent financial losses associated with dishonest claims.
This translates into a more resilient and profitable insurance business.
Impact of AI on Underwriting
Aspect | Impact of AI |
---|---|
Speed | Significantly faster decision-making, leading to quicker policy approvals and enhanced customer satisfaction. |
Accuracy | Potentially more accurate risk assessment, leading to more informed underwriting decisions and better risk management. |
Cost | Reduced operational costs through automation and reduced reliance on human labor, potentially leading to increased profitability. |
Efficiency | Improved overall efficiency through streamlined processes, reduced errors, and faster turnaround times. |
Challenges and Limitations of AI-powered Underwriting
AI-powered underwriting promises efficiency and accuracy, but its implementation isn’t without hurdles. Understanding these challenges is crucial for successful integration and responsible deployment. Potential pitfalls in data quality, bias, and regulatory compliance must be addressed proactively.AI underwriting systems, while powerful, aren’t foolproof. They rely heavily on data, and if that data is flawed, the system’s output will be compromised.
Furthermore, inherent biases within the data can be amplified by AI algorithms, leading to unfair or inaccurate assessments. Regulatory compliance and ethical considerations are paramount, and the potential for algorithmic bias necessitates mitigation strategies.
Data Quality and Bias in AI Underwriting
The accuracy of AI underwriting hinges on the quality and representativeness of the training data. Incomplete, inconsistent, or inaccurate data can lead to flawed models, resulting in incorrect risk assessments and potentially costly errors. For example, if a dataset lacks representation from diverse demographics, the AI model might not accurately assess the risk for certain groups, perpetuating existing biases.
Ensuring data diversity and thorough validation are essential steps in mitigating these risks.
Regulatory Compliance and Ethical Considerations
AI underwriting systems must adhere to stringent regulatory requirements. Transparency and explainability of the AI models are crucial to ensure compliance and trust. Regulations regarding data privacy, security, and fairness must be rigorously followed to avoid legal issues. Ethical considerations include the potential for discrimination and the need for oversight to prevent bias from negatively impacting specific demographics.
Algorithmic Bias and Mitigation Strategies
AI algorithms, trained on existing data, can inadvertently perpetuate existing societal biases. This can manifest in unequal risk assessments for different demographic groups. For example, if historical data reflects a higher rate of defaults for a specific ethnicity, an AI model might amplify this bias, leading to discriminatory lending practices. Mitigation strategies include regular audits of the models, continuous monitoring for bias, and employing diverse teams to develop and review the AI systems.
Comparison of AI Algorithms in Underwriting
Algorithm | Strengths | Weaknesses |
---|---|---|
Logistic Regression | Simple, interpretable, relatively fast | May not capture complex relationships, less powerful than more advanced models |
Decision Trees | Easy to understand, robust to outliers | Prone to overfitting, may not generalize well to new data |
Support Vector Machines (SVM) | Effective in high-dimensional spaces, good for classification | Computational cost can be high, less interpretable than other models |
Neural Networks | High accuracy in complex tasks, can learn intricate relationships | Difficult to interpret, prone to overfitting, requires significant data |
This table provides a simplified comparison of different AI algorithms. The choice of algorithm depends on the specific requirements of the underwriting process, the available data, and the desired level of accuracy and interpretability. It’s crucial to consider the trade-offs between these factors when selecting an algorithm for a particular application.
The Future of AI-powered Underwriting
AI is rapidly transforming the insurance industry, and underwriting is no exception. The integration of artificial intelligence promises to revolutionize how insurance companies assess risk, price policies, and manage claims. This evolution is driven by the potential for greater accuracy, efficiency, and personalization in the underwriting process.The future of AI in underwriting is poised to bring about significant changes in the insurance landscape.
From automating complex calculations to predicting future risks, AI’s capabilities are opening up new possibilities for insurers. This includes the development of more sophisticated risk models, leading to better pricing and risk management strategies.
Personalized Pricing Models
AI’s ability to analyze vast amounts of data allows for the creation of highly personalized pricing models. These models can consider individual factors, such as driving habits, health data, and even lifestyle choices, to tailor premiums. For instance, a telematics-based insurance policy using AI can adjust premiums based on a driver’s real-time driving behavior, rewarding safe drivers with lower rates.
This level of granularity in pricing is expected to improve customer satisfaction and increase profitability for insurers.
Dynamic Risk Management
AI can dynamically adjust risk management strategies based on real-time data. By monitoring various factors influencing risk, such as weather patterns, social trends, or even news events, AI can proactively adjust coverage and pricing. This adaptive approach is crucial in mitigating potential losses and ensuring the financial stability of the insurance company. An example is using AI to assess flood risk in real-time and adjust flood insurance premiums in affected areas.
Integration with Emerging Technologies
The future of AI-powered underwriting extends beyond traditional insurance models. AI is expected to seamlessly integrate with other emerging technologies like blockchain, the Internet of Things (IoT), and cloud computing. Blockchain can enhance the security and transparency of policy transactions, while IoT devices can provide valuable data on usage patterns and risk assessment. Cloud computing can facilitate the storage and processing of the massive datasets required for AI-driven underwriting.
Projected Growth and Adoption of AI in Underwriting (Next 5 Years)
Year | Projected Adoption Rate (%) | Key Developments |
---|---|---|
2024 | 25% | Initial widespread adoption of AI-powered risk assessment tools in select segments. |
2025 | 40% | Increased use of AI in personalized pricing and dynamic risk management. |
2026 | 60% | Integration of AI with other emerging technologies, like blockchain and IoT, becomes more common. |
2027 | 75% | Significant improvements in accuracy and efficiency of AI underwriting models. |
2028 | 90% | AI-driven underwriting becomes the standard practice for most insurers. |
AI’s evolution in underwriting is expected to lead to a more efficient, personalized, and risk-aware insurance industry.
Case Studies and Examples of AI in Underwriting
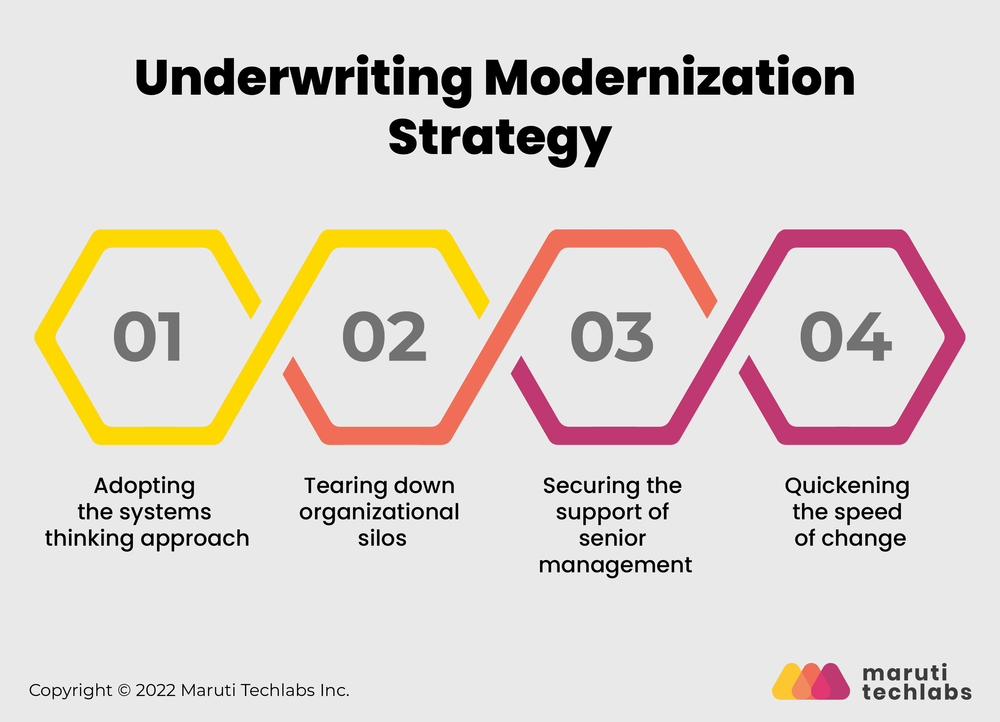
Source: marutitech.com
AI is rapidly transforming the underwriting process, offering potential for increased efficiency, accuracy, and fairness. This section examines real-world examples of how companies are using AI to assess risk and make underwriting decisions, highlighting both successes and challenges.AI algorithms can analyze vast amounts of data, identifying patterns and insights that might be missed by human underwriters. This allows for more personalized and data-driven risk assessments, potentially leading to more accurate and efficient underwriting decisions.
Specific Examples of AI in Underwriting
Several companies are already leveraging AI to enhance their underwriting procedures. These implementations demonstrate the diverse applications of AI across different insurance sectors.
- Auto Insurance Companies: Many auto insurance providers are utilizing AI to analyze driving data from telematics devices, such as those embedded in vehicles. These companies can use the data to assess risk more precisely, identifying drivers with lower accident rates and offering them lower premiums. For instance, some companies use machine learning to evaluate factors like driving style, location, and time of day to tailor coverage and pricing.
AI-powered underwriting is becoming increasingly important in finance. It’s helping streamline the process, and with the rise of Banking-as-a-Service (BaaS), Banking-as-a-Service (BaaS) providers are leveraging these tools to offer more flexible and personalized financial services. This ultimately boosts the efficiency of AI-powered underwriting in a wider range of financial applications.
- Property Insurance Companies: AI can analyze data about property characteristics, location, and historical claims data to predict the likelihood of future claims. This allows insurers to adjust premiums accordingly and identify properties with a higher risk profile. Examples include algorithms that analyze building designs and construction materials to determine potential vulnerabilities and predict potential damages from natural disasters.
- Life Insurance Companies: AI is being explored in the life insurance sector to assess health risk more accurately. This can involve analyzing medical records, genetic data, and lifestyle factors to estimate mortality risk more effectively. Some companies use AI-powered tools to identify potential health issues in applicants that might not be apparent from standard medical examinations.
Success Factors in AI Underwriting
The successful implementation of AI in underwriting hinges on several key factors.
- Data Quality and Availability: High-quality data is crucial for training accurate AI models. Insurers need to ensure the data they use is comprehensive, consistent, and free from bias. This often involves significant data cleaning and preparation processes.
- Model Transparency and Explainability: Understanding how AI models arrive at their decisions is vital for building trust and ensuring fairness. “Black box” models can raise concerns about bias or discrimination. Explainable AI (XAI) techniques are gaining traction to address this issue.
- Regulatory Compliance: Insurers must comply with all relevant regulations and guidelines when using AI in underwriting. This includes privacy laws, anti-discrimination laws, and financial regulations. Ensuring compliance from the outset is essential.
Companies Using AI in Underwriting
While specific companies often keep details of their AI implementations confidential for competitive reasons, here are some examples of firms known for using AI in various stages of their underwriting processes:
- AIG
- Progressive
- MetLife
- State Farm
- Liberty Mutual
Regulatory and Ethical Considerations in AI Underwriting
AI-powered underwriting is rapidly changing the insurance industry, but its implementation raises important regulatory and ethical concerns. Careful consideration of these factors is crucial for ensuring fair, equitable, and responsible use of AI in this critical process. These considerations are vital for building trust and maintaining public confidence in the insurance sector.
Importance of Regulatory Compliance
Regulatory compliance is paramount in AI-powered underwriting to ensure that AI systems adhere to existing laws and regulations. Failure to comply can result in significant penalties and reputational damage for insurers. This involves adhering to consumer protection laws, data privacy regulations, and anti-discrimination mandates. Understanding and anticipating future regulatory changes is also critical. Insurers must stay informed about emerging regulations and adapt their AI systems accordingly.
This proactive approach safeguards against potential legal issues and ensures the system operates within the boundaries of the law.
Ethical Considerations in AI Decision-Making, AI-powered underwriting
Ethical considerations in AI underwriting go beyond simple compliance. The potential for AI to perpetuate existing societal biases or create new ones requires careful consideration. Insurers must strive for fairness, transparency, and accountability in their AI-powered underwriting processes. This includes a deep understanding of how AI systems make decisions and what factors influence these decisions. Promoting fairness and minimizing potential harm to individuals is essential.
AI-powered underwriting is becoming increasingly sophisticated, using complex algorithms to assess risk. A key part of this process is the use of tokenization, which helps protect sensitive data while enabling smooth processing. This process of tokenization ( Tokenization ) is crucial for accurate risk assessment in the underwriting process, ultimately improving the efficiency and security of AI-powered underwriting systems.
Potential for Bias and Discrimination in AI Algorithms
AI algorithms, trained on historical data, can inherit and amplify existing societal biases. For example, if historical data reflects gender or racial disparities in insurance claims, the AI system may perpetuate these biases in its underwriting decisions. This could lead to discriminatory outcomes, impacting access to insurance for certain groups. To mitigate this risk, insurers must carefully examine the data they use to train their AI models and actively seek ways to address any biases present.
Insurers need robust mechanisms to identify and mitigate potential biases, ensuring fairness and equity for all customers.
Importance of Transparency and Explainability in AI Systems
Transparency and explainability are critical for building trust and accountability in AI-powered underwriting. Customers need to understand how AI systems make decisions about their applications. When an AI system denies a claim or assesses a premium, it’s essential that the customer can understand the reasons behind the decision. This involves developing AI systems that provide clear explanations for their outputs.
AI-powered underwriting is becoming increasingly important in the digital finance world. It’s streamlining the entire process, from application to approval, by automating many tasks and using algorithms to analyze risk more effectively. This leads to faster and more efficient decisions, a key component of modern Digital finance solutions. Ultimately, AI-powered underwriting helps create a more accessible and responsive financial system for everyone.
This not only fosters trust but also facilitates the ability to identify and correct any errors or biases in the system.
Legal and Ethical Frameworks for AI in Underwriting
Developing a robust legal and ethical framework for AI in underwriting requires a multi-faceted approach. Insurers should collaborate with policymakers, regulators, and legal experts to establish clear guidelines and standards. These frameworks should cover data privacy, algorithmic fairness, transparency, and accountability. They should also anticipate future developments in AI technology and address any emerging challenges. A comprehensive approach is needed to ensure the responsible and ethical use of AI in the insurance industry.
AI Underwriting in Specific Insurance Sectors: AI-powered Underwriting
AI-powered underwriting is rapidly transforming the insurance industry, and its application varies significantly across different insurance sectors. The unique characteristics of each sector, from risk assessment to policy design, dictate how AI is deployed and the specific challenges encountered. Understanding these sector-specific applications is crucial to appreciating the full potential and limitations of AI in insurance.
Auto Insurance
Auto insurance, with its focus on vehicle-related risks, presents a fertile ground for AI applications. AI can analyze vast datasets of driving behavior, accident records, and vehicle characteristics to assess risk more accurately than traditional methods. This allows for personalized premiums and tailored coverage options, enhancing customer experience and profitability.
AI-powered underwriting is becoming increasingly sophisticated, automating many aspects of the process. This can lead to faster approvals and more efficient operations, but also raises some interesting questions about risk assessment in a rapidly changing financial landscape, particularly with the rise of Stablecoins. Ultimately, though, the goal remains the same: ensuring sound underwriting practices for a stable financial system.
- Predictive modeling of accident risk based on driver behavior, vehicle type, and location data is a key application. This allows insurers to identify high-risk drivers and offer customized premiums, potentially leading to significant cost savings.
- Usage-based insurance (UBI) is gaining traction. AI algorithms can track driving patterns in real-time, providing personalized premiums based on safe driving habits.
- Fraud detection is another crucial application. AI algorithms can analyze claims data to identify patterns indicative of fraudulent activity, preventing financial losses.
Home Insurance
AI can also enhance home insurance underwriting by analyzing factors like property value, location, building materials, and even weather patterns. This enables insurers to offer accurate risk assessments and adjust premiums accordingly.
- Risk assessment is crucial. AI algorithms can assess factors like proximity to natural disasters, building quality, and security measures, creating personalized risk profiles for homeowners.
- Claims analysis can be automated, allowing insurers to process claims more efficiently and identify potential fraud.
- Personalized pricing based on the unique characteristics of each home is a significant opportunity, fostering better customer relationships and increasing profitability.
Life Insurance
AI’s role in life insurance is multifaceted, focusing on longevity predictions, assessing health risks, and personalized policy offerings.
- Longevity prediction based on factors like genetics, lifestyle, and medical history is a powerful application. AI can help predict life expectancy and calculate premiums based on an individual’s projected lifespan.
- Personalized policy design is a key element, tailoring coverage and premiums to individual needs and risk profiles.
- Health risk assessment, leveraging wearable data and medical records, is a potential game-changer, allowing insurers to offer more accurate and targeted coverage options.
Comparison of AI in Different Product Lines
The application of AI in different insurance product lines varies. While auto insurance benefits from UBI and predictive modeling, life insurance relies heavily on longevity prediction and health risk assessment. Home insurance leverages data on property characteristics and risk factors. The specific algorithms and data utilized differ based on the unique risks and characteristics of each product line.
Final Conclusion
In conclusion, AI-powered underwriting is rapidly changing the insurance landscape. While it presents significant opportunities for increased efficiency, accuracy, and reduced bias, careful consideration of ethical and regulatory implications is crucial. Future advancements in AI could lead to personalized pricing and dynamic risk management, offering a more tailored and responsive insurance experience. However, the success of AI in underwriting depends on mitigating bias, ensuring regulatory compliance, and building trust with consumers.
FAQ Resource
What are some common data sources used in AI-powered underwriting?
AI underwriting leverages a wide range of data, including historical claims data, credit reports, social media activity (with privacy considerations), and even driving behavior. The more comprehensive and varied the data, the more accurate the risk assessment tends to be.
How does AI mitigate bias in underwriting?
AI algorithms can be trained to identify and account for existing biases in historical data. Ongoing monitoring and auditing are essential to prevent any unintended bias from creeping into the system. Regular updates and recalibration of the algorithms can help keep the process fair.
What are the biggest concerns about AI bias in underwriting?
Concerns include the potential for algorithms to perpetuate existing societal biases if not carefully designed and monitored. Also, ensuring the data used to train these models is representative and free of bias is vital.